Can AI uncover the laws of physics by observing apples falling from trees?
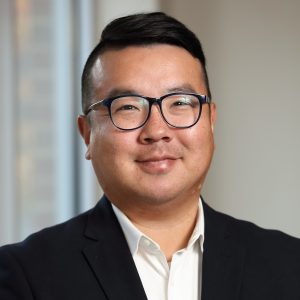
Date: Friday, Apr 18, 2025
Start time: 2:00 pm
Location: 701 W. Grace St., Room 2306
Audience: All are welcome to attend.
Prof. Stephen S. Baek
School of Data Science
University of Virginia
Abstract
Machine learning is playing an increasingly vital role in the domain of physics simulation. On this evolving frontier is so-called physics-aware deep learning (PADL). Unlike conventional deep neural networks that focus on input-output mapping, PADL algorithms can make accurate predictions of physical phenomena, while adhering to physical principles and constraints. So far, on relatively simple spatiotemporal dynamics problems, such as predicting vortex shedding in Navier-Stokes simulations, the prediction accuracy and speed of PADL algorithms have been demonstrated. However, when it comes to problems with strong nonlinearity, characterized by sharp gradients, fast-transient features, discontinuous evolving boundaries, etc., common in many real-world problems, PADL’s effectiveness still remains unclear. Particularly, from a purely statistical perspective, these extreme features are viewed as “statistical outliers” that must be disregarded to prevent overfitting; while from the physics standpoint, these are in fact the most important characteristics that must be modeled precisely. Such a gap between the statistical view of model training and the scientific needs for predicting nonlinear features renders an interesting opportunity for transdisciplinary research, which has been the main focus of my research team lately. In this talk, I will provide an overview of the mathematical and computational challenges in applying modern deep learning models for solving real-world physics problems. I will then introduce our recent work called physics-aware
recurrent convolutional neural networks (PARC) and show its effectiveness in modeling extreme physical phenomena. I will also compare PARC with other established PADL baselines and discuss current challenges and future directions.
Brief bio:
Dr. Stephen Baek is an applied geometer and data scientist with a keen interest in
geometric data analysis. He earned his bachelor’s and PhD degree in Mechanical and Aerospace Engineering from Seoul National University, Korea. He was a postdoctoral researcher at the Institute for Advanced Machinery Design in Seoul National University. Dr. Baek was the recipient of the National Science and Engineering Scholarship and the Global Ph.D. Fellowship from the Korean Ministry of Education, and the Presidential Postdoc Fellowship from the President of the Republic of Korea. Currently, he is a Quantitative Foundation Associate Professor of Data Science at UVA. He has been leading various research projects sponsored by Air Force, Army, DOE, NSF, NIH, NASA, DOT, Hyundai Motors, and NFL.
Event contact: Denis Demchenko, ddemchenko@vcu.edu