Physics Colloquium: Ka Un Lao, Ph.D.
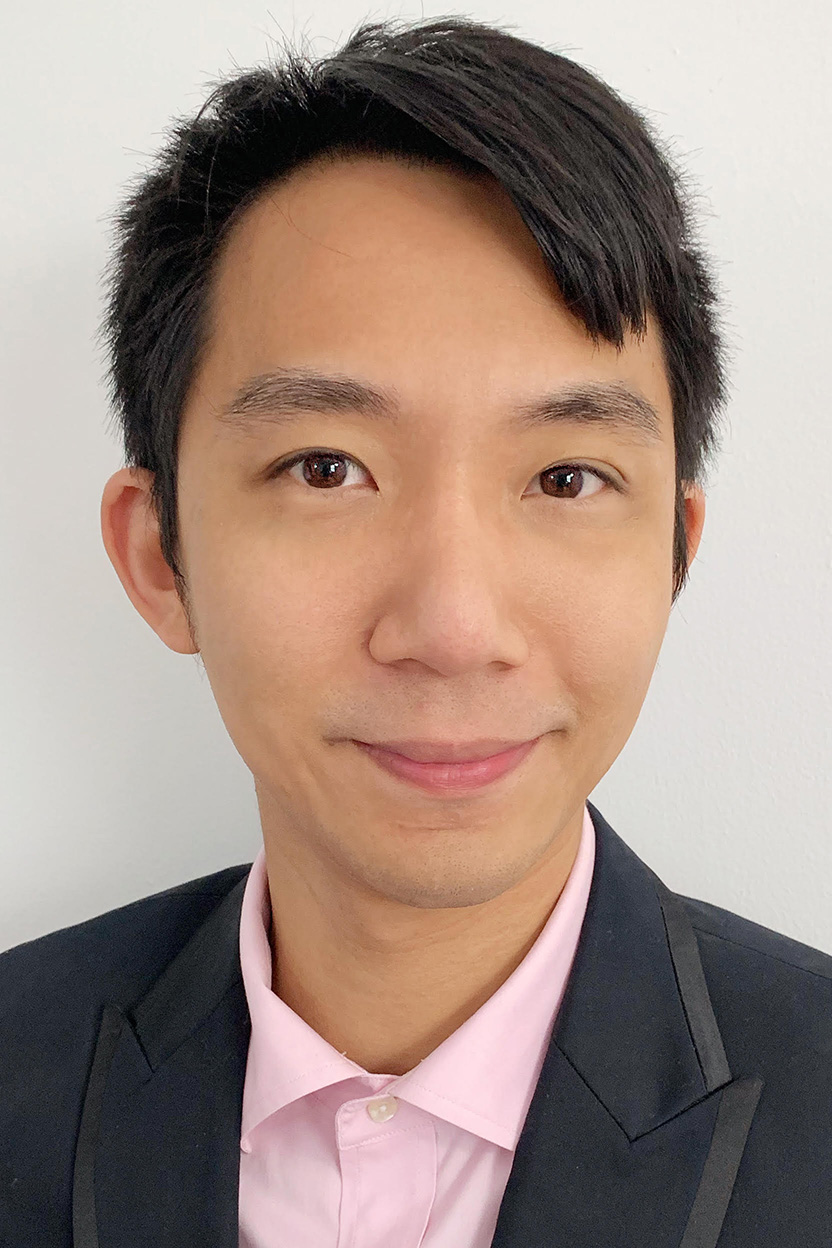
Date: Friday, Feb 23, 2024
Location: 701 W. Grace St., Room 2306
Accelerating Quantum Chemistry Calculations Using Grassmannians, Fragmentations, and Machine Learning
Quantum chemistry (QC) has significantly advanced research in modern chemistry, allowing the study of chemical properties and processes at the quantum scale. However, accurate QC methods often require substantial computational resources and time, particularly for large and complex systems. Our group has developed three innovative QC tools to address this computational bottleneck, enabling faster research progress than ever before. The first tool integrates Grassmannian mathematics from differential geometry with electronic structure theory, enabling the efficient calculation of high-quality density matrices at each point on potential energy surfaces. This approach substantially accelerates or even eliminates the time-consuming self-consistent field iterative procedure commonly used in density functional theory. Another tool to overcome the nonlinear scaling of QC methods is the fragmentation approach. Our group has devised an accurate and efficient GMBE-DM fragmentation scheme based on set theory. It demonstrates highly accurate absolute and relative energies across a wide range of systems, outperforming other fragmentation approaches by an order of magnitude. Moreover, GMBE-DM achieves these results with faster computational speed, without requiring significant parallelization. Lastly, we have developed accurate and efficient machine learning (ML) models for predicting ab initio dispersion potentials, utilizing only Cartesian coordinates as input. This ML model can be integrated with MP2 to create the most accurate N5 scaling waverfunction method, suitable for modeling even large noncovalent complexes. Together, these three QC tools enable accurate and efficient calculations, significantly expanding the scope of systems amenable to electronic structure calculations. They make large systems and long-time scale simulations accessible within a fraction of the original computing time, thereby accelerating scientific discovery in quantum chemistry.
Speaker bio: Ka Un Lao was born in Macau and completed his B.Sc. and M.Sc. degrees in chemistry from National Tsing Hua University in Hsinchu, Taiwan, before pursuing his Ph.D. in theoretical chemistry at The Ohio State University, where he worked with John Herbert on fragmentation approaches and noncovalent interactions, graduating in 2016. From 2016 to 2019, he served as a postdoctoral associate at Cornell University, working with Robert DiStasio Jr. on electronic structure theory and machine learning for molecular properties. Since 2019, he has been an Assistant Professor in the Chemistry Department at Virginia Commonwealth University. His research interests encompass fragmentation quantum chemistry approaches, Grassmannians in electronic structure theory, intermolecular interactions and machine learning.
Event contact: Physics Department, physics@vcu.edu